Advanced techniques for fertility treatment
New technologies are getting enhanced everyday to shatter the thought of infertility and ensure the potential results of fertility by exploring the root cause of it.
At PSFC, we always thrive to hand out treatments which are equipped with the latest technology for providing services. By investing in innovative technologies, we always get the leading evidence in getting positive results from the client’s side.
With these services, it helps in increasing the chances of experiencing successful fertilization, implantation, conception and healthy pregnancy. In addition to improving the chances of success, these enhancements can reduce the chances of miscarriage which may happen by genetic complications.
But remember that these treatments are not necessary for every fertility patient, but they are critical for healthy outcomes when our specialists recommend them for your specific situation.
Embryo Selection
Artificial intelligence has gained traction in predicting clinical outcomes using acquired information, such as patient attributes, medical images, and blood test results. As a result, adopting Artificial Intelligence in IVF within the fertility clinic could significantly increase IVF success.
AI could aid the embryologist in providing a rapid, objective, and accurate assessment of gamete and embryo health. AI may also assist physicians in formulating an optimal, personalized fertility treatment plan based on patient characteristics.
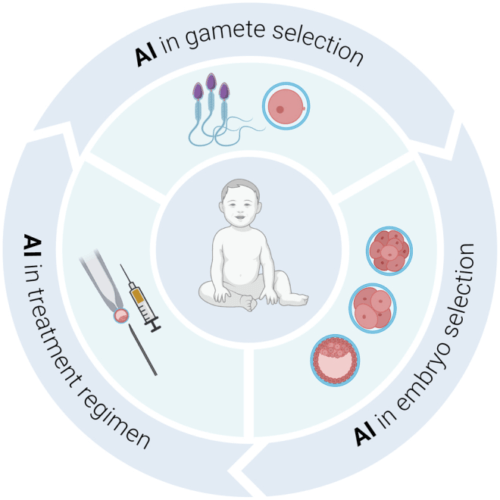
AI in gamete selection
Current clinical assessment of gamete health focuses on identifying early quality markers. This includes the visualisation of gametes through direct inspection, static images, or time-lapse videos. The quality of female gametes is associated with follicle size, oocyte morphology, and cytoplasmic characteristics. In terms of sperm, morphology, concentration, and motility are known factors directly correlated with IVF success. However, the selection is prone to high variation between operators. AI can be transformative in this aspect. AI would remove the subjectivity of human assessment from the decision-making process and objectively rank gametes based on quality.
However, the use of AI for oocyte selection may be limited due to the practice of fertilising all available oocytes unless AI could predict blastocyst formation or, more importantly, live birth before fertilisation. The most significant benefit of AI may come from the selection of sperm for intracytoplasmic sperm injection (ICSI), a process currently performed by embryologists. The development of new assessment criteria for sperm selection might arise through unsupervised AI, where new markers of sperm quality are identified, such as swimming patterns, the direction of motion, or differences in sperm compartments (i.e. length of head vs tail). Although more challenging, AI may compute the optimal sperm–egg combination to achieve the highest success rate or determine whether IVF or ICSI is the best fertilisation approach.
Interestingly, AI has demonstrated proficiency in predicting fertility outcomes based on distinct ultrastructural details of mouse sperm – a larger sperm head compared to the midpiece is associated with improved blastocyst development. Similarly, AI has yielded high accuracy in classifying human sperm using kinetic parameters – 89.9%; as well as classifying sperm head morphology with a high concordance rate with current manual classifications (SCIAN and HuSHeM, 88 and 94%, respectively). Notably, these studies used images or videos acquired during a routine assessment. AI can, therefore, complement current clinical practices whilst offering objective gamete selection to substantiate the evaluation made by embryologists.
AI in embryo selection
Morphological assessment of embryos is the most commonly used process to select embryos for transfer. It occurs through direct visualisation using a light microscope or by time-lapse imaging. Both approaches grade embryos on their ability to reach particular stages of development on time. As with gamete selection, there is a high degree of variation between operators and clinics due to the subjective nature of these assessments. Consequently, standardisation is challenging within a clinic and near-impossible between institutions.
As such, morphological grading remains limited in predicting live birth outcomes. However, Fertility Centre in Chennai, using AI routinely generated images or time-lapse videos, may objectively and accurately grade and rank embryos, thus assisting in the decision-making process to transfer or freeze them. Further, Artificial Intelligence Embryo Selection may have a role in analysing data from non-invasive metabolomic and secretory profiles from the embryo during culture. Consequently, this may lead to improved culture media formulations and regimens.
AI in treatment regimen
In the IVF Centre in Chennai, decision-making for an IVF cycle regimen is guided by patient age, gamete quality, medical history, and many more. This process intends to maximise the chances of pregnancy and the birth of a healthy baby. From patient to patient, an IVF cycle might thus differ in stimulation protocol and mode of fertilisation (IVF vs intracytoplasmic sperm injection) and the potential for other procedures, including assisted hatching and preimplantation genetic testing, amongst others.
Planning for an IVF cycle relies heavily on input from the clinician, who may prescribe a different treatment regimen based on their own clinical experience and clinic-to-clinic differences in training and in-house practice. AI could aid fertility practitioners in this aspect, enabling objective decision-making to optimise the treatment protocol for the best outcome. In addition, AI applies to data-mining patient records to discover novel markers predicting pregnancy and live birth.
Why does reproductive medicine require AI?
The rapid development of ART, such as oocyte and embryo cryopreservation, assisted fertilization, preimplantation genetic testing and embryo selection technologies, have greatly improved the clinical pregnancy rate in the 40 years since the first in vitro fertilization (IVF) babies, even though many problems remain. The quality of embryos is the most critical factor for the success of IVF, but there is still a lack of methods of judging the quality of the eggs, the sperm and the embryos accurately. In addition, ET In IVF methods using a single parameter or algorithm have yet to be identified. Therefore, it is difficult to predict the probability of a successful pregnancy for each patient and fully understand each failure’s cause.
AI-based methods in reproductive medicine may become a solution to current dilemmas. The primary driver for developing these applications is the desire to improve the treatment and prognosis for infertility patients, using the large quantities of data provided by complex diagnostic and therapeutic modalities. AI can provide greater efficacy and efficiency in clinical activities, thereby optimizing the treatment cycle of ART.